Artificial intelligence continues to dominate headlines, benchmark tests, and boardroom discussions. But while flashy demos and high scores on academic datasets impress the media, one question remains underexplored: Can AI actually execute real-world work from end to end? Or are we mistaking isolated cognitive tasks for full-spectrum intelligence?
This article explores a critical distinction in both human and machine capabilities: the gap between knowing something and being able to do something with it. We’ll examine the three types of human skillsets, how AI maps to these categories, and why the often-overlooked role of the human “integrator” remains essential in any AI-driven workplace.
The Three Types of Human Intelligence
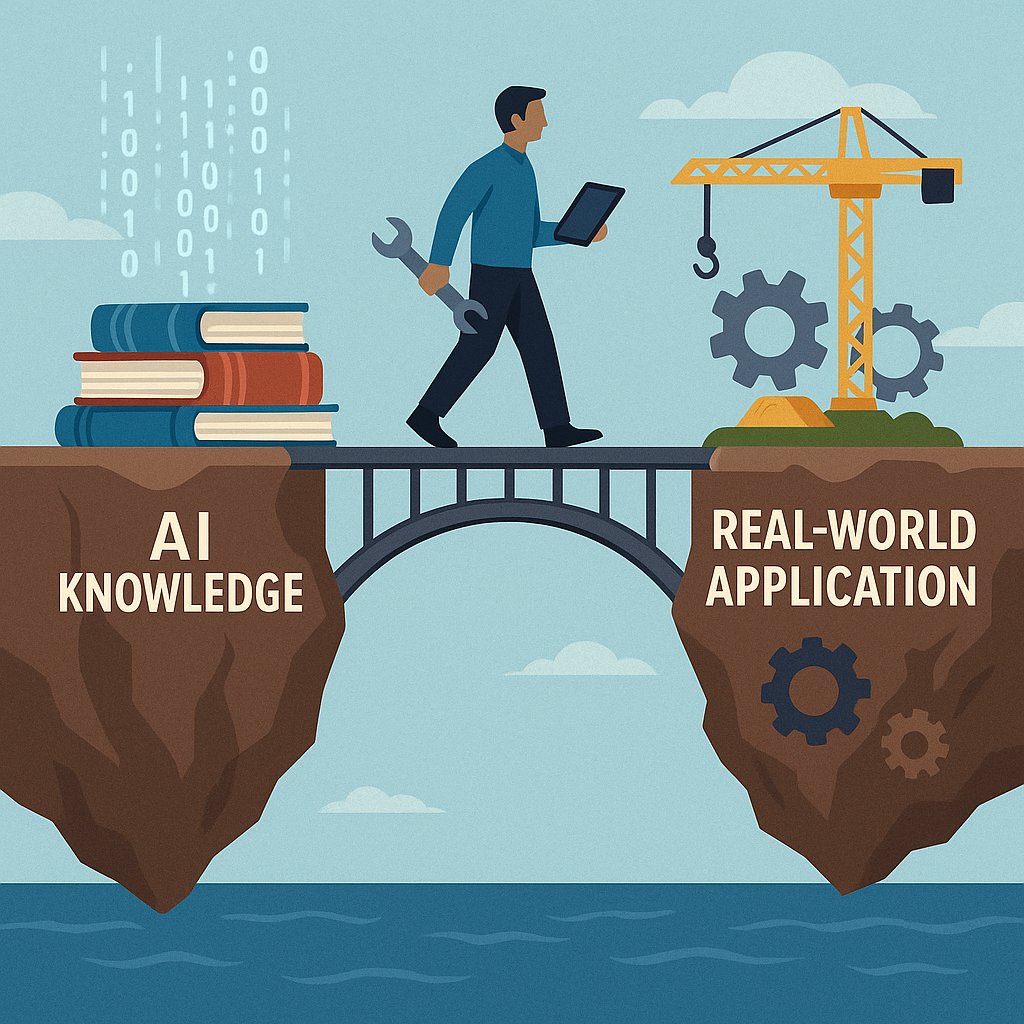
While people rarely fall cleanly into one bucket, we can generally describe three distinct types of human intelligence in the workplace:
- Book Smart – These are the people with encyclopedic knowledge. They ace tests, can recall definitions, and often know the theory behind how things work. But they may struggle when asked to translate that knowledge into practical action.
- Practical Smart – These individuals are grounded, hands-on, and can get real work done. They may not know the theoretical foundations, but they can repair an engine, build a deck, or debug a production system through experience.
- Integrators – The rare but crucial group who can understand complex theories and make them usable. These people serve as bridges between academic knowledge and practical implementation. Think of them as the translators between R&D and operations, or between AI researchers and real-world application teams.
Where AI Succeeds — and Fails — on This Spectrum
AI is often benchmarked against book smart tasks:
- Passing standardized tests (e.g., bar exams, SAT)
- Writing essays
- Coding small functions
- Answering trivia
These tasks are impressive, but limited. Current AI does not demonstrate:
- Mechanical improvisation
- Cross-domain coordination
- Judgment in open-ended, uncertain environments
In other words, today’s AI performs like a very fast book-smart intern — capable of helping on tightly-scoped tasks, but unable to take full ownership of real-world processes.
Real-World Work Is Messy and Integrated
Imagine telling a human-like robot:
We have a new product launching in Department 42. Go design, build, test, and ship the machinery to assemble, package, and quality check that product.
AI isn’t there. It doesn’t possess the real-world context, hands-on coordination, or judgment to:
- Talk to the line workers
- Find missing tools
- Adjust the design for vibration issues
- Troubleshoot electrical interference
We do have AI-driven robots in factories — but they paint, weld, or pick parts. They’re specialized, not autonomous managers or designers. They don’t understand the why, just the how (as programmed by humans).
The Forgotten Role of the Integrator
In both human organizations and AI development, integrators are the bottleneck and the key.
- They understand enough of the AI model’s logic.
- They understand the frontline process, constraints, and people.
- They can translate abstract outputs into process change.
Most failed AI projects don’t fail because the model was bad — they fail because nobody bridged the gap between the lab and the loading dock. The integrator role is the glue that AI systems still can’t replace.
Misleading AI Comparisons and Tests
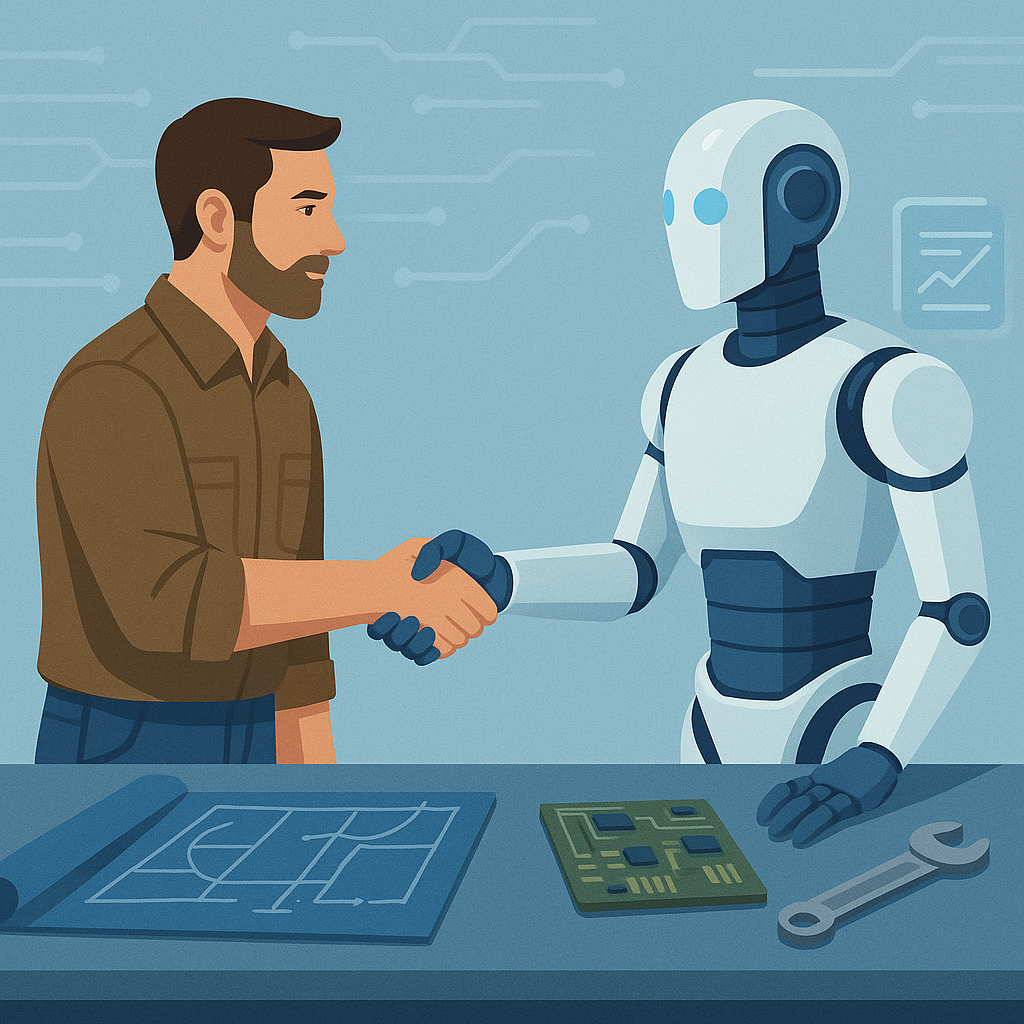
The Turing Test, MMLU scores, and HumanEval datasets test specific abilities — not full workflows. They don’t reflect how AI behaves across months of work, under changing requirements, within teams, or amid real-world friction.
Just like being “book smart” doesn’t mean you can build a house, passing an AI benchmark doesn’t mean an AI system can deliver business value unaided.
Conclusion: Respect the Bridge Between AI and Real-World Application
AI is an incredible tool — but tools require craftsmen. While language models, robots, and narrow AI systems get more powerful, they still fall short of executing real-world integration. That work still belongs to humans who combine theory with judgment, constraints with opportunity, and vision with delivery.
In the future, the most valuable professionals won’t be those who fear AI or those who blindly worship it. They’ll be the ones who can integrate it — practically, creatively, and wisely.
Explore the free Infographic
See our companion infographic, “Reality Check: AI vs Human Practical Intelligence,” for a visual breakdown of this spectrum. Please share with friends, family, and coworkers.
Reality Check: AI vs Human Practical Intelligence
Want to stay ahead in applied AI?
📑 Access Free AI Resources:
- Download our free AI whitepapers to explore cutting-edge AI applications in business.
- Check out our free AI infographics for quick, digestible AI insights.
- Explore our books on AI and .NET to dive deeper into AI-driven development.
- Stay informed by signing up for our free weekly newsletter